Abstraction in RL
ICML WORKSHOP 2016

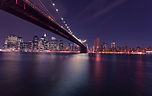
New York,
23rd June 2016
CALL FOR PAPERS
The Abstraction in Reinforcement Learning Workshop will be held on the 23rd June 2016 in New York, USA.
Submission Deadline: 1st May 2016, 11:59 PM (GMT+2)
Submission URL: https://cmt3.research.microsoft.com/ARL2016/
We will be accepting extended abstracts of between 4-6 pages (including references). We encourage authors to send works that are currently in progress if they feel that this workshop will provide a good platform for discussing the caveats or advantages of their approach.
Paper submissions need to be based on one of the following topics:
Abstraction Representations: New, Interesting and scalable abstraction representations that can be advantageous for current state-of-the-art RL algorithms.
Learning Abstractions: Novel approaches or frameworks for learning abstractions in Reinforcement Learning (RL). These approaches can deal with any relevant form of RL abstraction. Examples include temporally extended actions such as options or skills as well as spatial abstractions such as state space representations.
Algorithms: New scalable algorithms that make use of abstractions in order to perform planning and learning in RL.
Abstractions based on Deep Learning: With the recent emergence and relative success of Deep Reinforcement Learning algorithms such as Deep-Q Networks, papers that make use of Deep Learning algorithms to learn or represent RL abstractions are encouraged.
Benchmarks: We are always looking for exciting new benchmarks or frameworks for learning abstractions in RL. The suitability of these benchmarks to show-casing abstractions is really important. Therefore, we also expect the performance results of abstraction-based RL algorithms on these new domains.
Acceptance Criteria: Papers will be judged based on their novelty, relevance, technical signifance and clarity.
Sponsored by:
